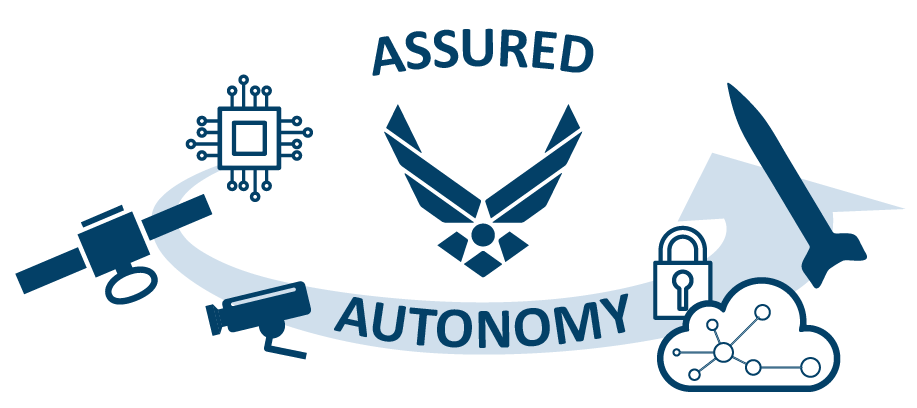
Spring 2021 AACE Seminar Series
-
Characterization of Satellite Swarms Under Non-Keplerian Dynamics for the Purposes of Assuring Autonomy,
Taryn J. Noone and Dr. Norman G. Fitz-Coy, University of Florida, January 20, 2021 Abstract: While the processes by which satellite swarms are defined, initialized, and propagated have been explored in the case of Keplerian dynamics, it has remained vital to assess the manner in which said processes are affected by the introduction of non-Keplerian disturbance terms. Such disturbances require active inputs to compensate for them, which poses a potentially limiting factor in the effectiveness and autonomy of the swarm. There carries a possibility that such disturbances may disrupt the integrity of the swarm, or dissolve it entirely, adversely affecting the ability of the swarm satellites to pass information between one another. Principally, this is accomplished using the Lagrange Planetary Equations (LPE) to characterize force-defined (e.g., a burst of thrust from an onboard engine) and acceleration-defined (e.g., acceleration due to the gravitational influence of the Sun or Moon) disturbance terms. Application of the LPE into the Swarm Cost Functional (SCF) will be used as a starting point for this analysis, which is intended to gain insight into how various disturbance forces affect the evolution of the swarm formation over time, as well as assess the required expenditure of delta-v for swarm maintenance.
-
Attitude and orbital spacecraft maneuvering in the presence of physical and environmental uncertainties,
Camilo Riaño-Ríos and Dr. Riccardo Bevilacqua, University of Florida, February 3, 2021 Abstract: The increasing number of applications for swarms of small satellites motivates research efforts on the development of efficient methods for attitude and orbital maneuvering. These swarms provide versatility and robustness to the mission, but given the volume limitations of each agent, processing and propellant capabilities are often reduced. In this talk, recent results on propellant-less attitude and orbital maneuvering for small satellites in low earth orbit using aerodynamic drag and gravity gradient torque, are presented. The designed maneuvering algorithms apply adaptive control and integral concurrent learning to compensate for uncertain physical and environmental parameters and estimate them online. The next set of challenges to be addressed in this problem are discussed, and a new potential application for this control framework is also presented.
-
Distributed and Non-Stationary Zeroth-Order Reinforcement Learning,
Yan Zhang and Dr. Michael Zavlanos, Duke University, February 17, 2021 Abstract: Reinforcement learning (RL) has been widely used to solve sequential decision making problems in unknown stochastic environments. In this talk we first present a new zeroth-order policy optimization method for Multi-Agent Reinforcement Learning (MARL) with partial state and action observations and for online learning in non-stationary environments. Zeroth-order optimization methods enable the optimization of black-box models that are available only in the form of input-output data and are common in training of Deep Neural Networks and RL. In the absence of input-output models, exact first or second order information (gradient or hessian) is unavailable and can not be used for optimization. Therefore, zeroth-order methods rely on input-output data to obtain approximations of the gradients that can be used as descent directions. In this talk, we present a new one-point policy gradient estimator that we have recently developed that requires a single function evaluation at each iteration to estimate the gradient, by using the residual between two consecutive feedback points. We refer to this scheme as residual feedback. We show that residual feedback in MARL allows the agents to compute the local policy gradients needed to update their local policy functions using local estimates of the global accumulated rewards. Also, in online learning, one-point policy gradient estimation is the only viable choice. We show that, in both MARL and online learning, residual feedback induces a smaller estimation variance than other one-point feedback methods and, therefore, improves the learning rate.
-
HyNTP: An Adaptive Hybrid Network Time Protocol for Clock Synchronization in Heterogeneous Distributed Systems,
Marcello Guarro and Dr. Ricardo Sanfelice, University of California Santa Cruz, March 3, 2021 Abstract: Clock synchronization over networks is a challenging problem that has long been an important topic in the fields of computer science and engineering as it pertains to digital networks and distributed systems. Recently, clock synchronization has received much attention in the study of networked control theory due to the importance of consensus on time in distributed control and estimation settings. Motivated by performance issues of existing synchronization protocols, we present a distributed hybrid algorithm that synchronizes the time and rate of a set of clocks connected over a network. Clock measurements of the nodes are given at aperiodic time instants and the controller at each node uses these measurements to achieve synchronization. Due to the continuous and impulsive nature of the clocks and the network, we introduce a hybrid system model to effectively capture the dynamics of the system and proposed hybrid algorithm. Moreover, the hybrid algorithm allows each agent to estimate the skew of its internal clock to allow for synchronization to a common timer rate. We provide sufficient conditions guaranteeing synchronization of the timers, exponentially fast. Numerical results illustrate the synchronization property induced by the proposed algorithm as well as robustness to communication noise.
-
Reinforcement Learning for Dynamic Spectrum Sharing,
Dr. John Shea, University of Florida, March 31, 2021 Abstract: The conventional approach to spectrum management, in which human experts create spectrum allocations for groups of users, are often inefficient for multiple reasons. They generally allocate frequency bands over relatively large geographic areas (tens to millions of $km^2$) for long periods of time (often days, months, or indefinitely). They are not adaptive to changes in the spatial distribution of users or the communication traffic. And in contested environments, they lock users into frequencies that may be subject to interference or jamming. Dynamic spectrum sharing (DSS) has the potential to overcome all of these issues by autonomously allocating spectrum among groups of users with adaptation on the order of seconds in response to changes in user distribution, channel conditions, communication traffic, and interference. These techniques can also provide very fine-grain spectrum adaptation over space (over $km^2$). However, DSS problems are inherently hard because of the huge number of parameters that characterize the DSS state space. In this talk, I will delve into DSS problems, discuss how we have chosen to decompose these problems to make optimization practical, and consider how reinforcement learning (RL) can be applied to one of the most fundamental DSS problems. Some results of applying RL are presented for a scenario in which five squads of soldiers and UAVs move in formation through an urban environment while having to handling increasing amounts of communication traffic.
-
Hard-label Manifolds: Unexpected Advantages of Query Efficiency for FindingOn-manifold Adversarial Examples,
Washington Garcia and Dr. Kevin Butler, University of Florida, April 14, 2021 Abstract: Designing deep networks robust to adversarial examples remains an open problem. Likewise, recent zeroth order hard-label attacks on image classification models have shown comparable performance to their first-order, gradient-level alternatives. It was recently shown in the gradient-level setting that regular adversarial examples leave the data manifold, while their on-manifold counterparts are in fact generalization errors. In this paper, we argue that query efficiency in the zeroth-order setting is connected to an adversary's traversal through the data manifold. To explain this behavior, we propose an information-theoretic argument based on a \textit{noisy manifold distance oracle}, which leaks manifold information through the adversary's gradient estimate. Through numerical experiments of manifold-gradient mutual information, we show this behavior acts as a function of the effective problem dimensionality and number of training points. On real-world datasets and multiple zeroth-order attacks using dimension-reduction, we observe the same universal behavior to produce samples closer to the data manifold. This results in up to two-fold decrease in the manifold distance measure, regardless of the model robustness. Our results suggest that taking the manifold-gradient mutual information into account can thus inform better robust model design in the future, and avoid leakage of the sensitive data manifold.